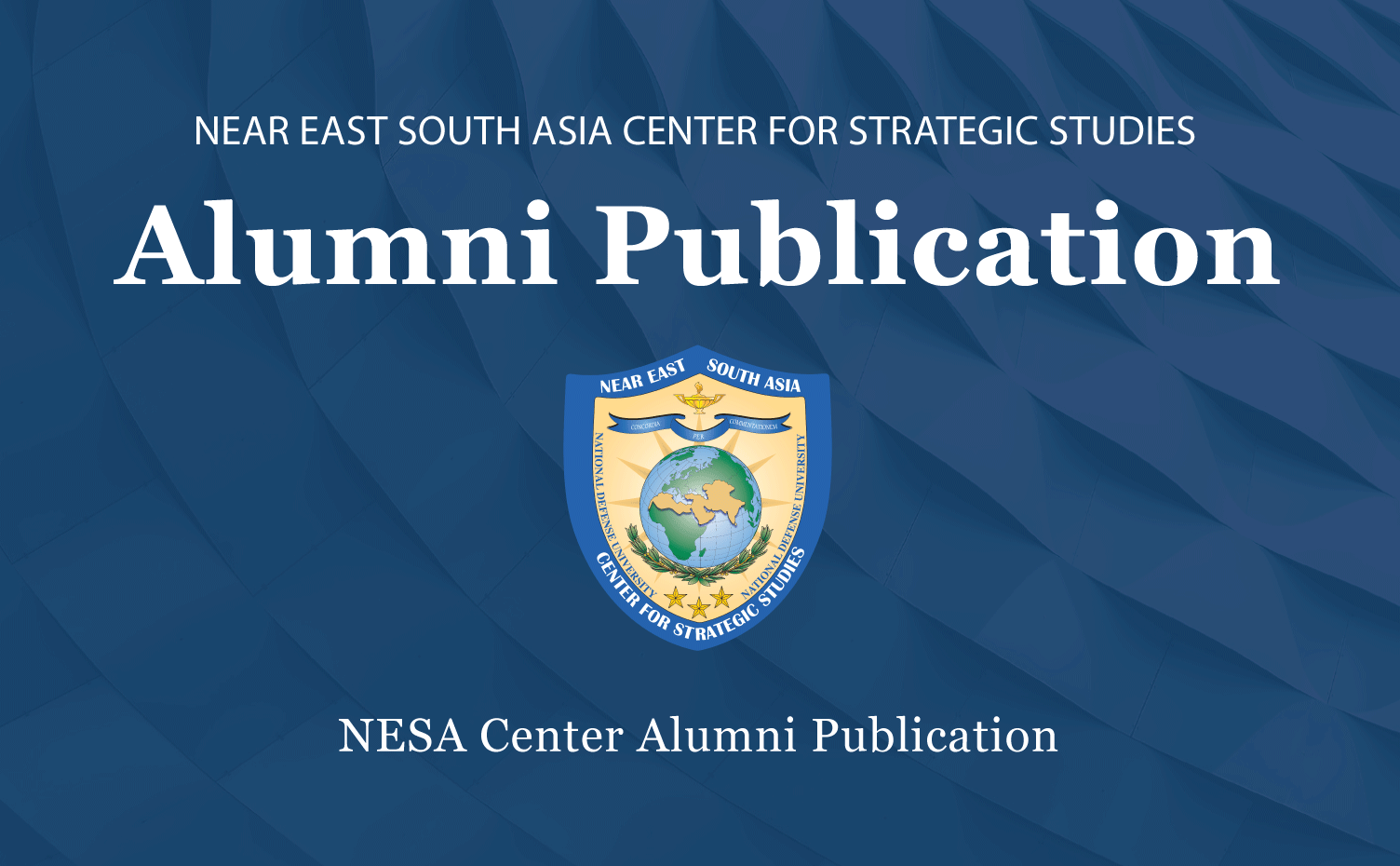
NESA Center Alumni Publication
Nimra Javed – NESA Center Alumnus serving as a Research Officer at the Center for International Strategic Studies AJK. Nimra holds an MPhil Degree in Strategic Studies from National Defence University, Islamabad.
3 February 2025
AI-driven strategies for counterterrorism and violent extremism hold immense potential due to AI’s ability to conduct predictive analysis, improve the surveillance capabilities by effectively utilizing existing resources, improving sensor- to- shooter link, and helping in removing extremist propaganda from social media. However, the success of these models depends on the factors such as training AI models in local languages, equipping them with cultural dynamics and providing them high-quality data so that these models can be effective in responding to the specific regional challenges.
Firstly, AI tools can help enhance the existing surveillance networks by analyzing their data and identifying threats by performing tasks that are humanly impossible and also not cost-effective. Modern surveillance networks such as cameras and sensors produce unprecedented amount of data. Due to the magnitude of data, it becomes impossible to analyze the data such as footage produced by surveillance cameras. Moreover, manually analyzing data can be prone to errors and also lack of speed makes it difficult to monitor activities in real-time. Due to strong analytical power of AI systems, they can identify patterns of behavior using various analytical techniques which are not apparent to human analysts.
Consider Islamabad’s Safe City project, which had over 1,950 cameras installed in 2016. The cameras are located on thoroughfares and in sensitive areas. There are only 125 screens at the Safe City Authority building for monitoring the cameras. However, if the Safe City Authority in Islamabad were to monitor footage of cameras installed, it would need substantial resources and manpower. Let’s assume an analyst can manage the footage from 10 cameras during the eight-hour shift. To meet this requirement, 195 analysts will be required to manage this workload in one shift. Continuous 24-hour monitoring would need 585 analysts working across three shifts. Assuming the minimum wage of 32000 for unskilled workers in Pakistan, merely paying the salaries of these analysts would cost 18.72 million rupees.
Surveillance Project Calculations
Description | Value |
Number of Cameras | 1950.0 |
Camera Hours per Day | 46800.0 |
Analysts per Shift | 195.0 |
Shifts per Day | 3.0 |
Total Analyst Shifts Needed | 585.0 |
Product of Minimum wage and Analysts | 18720000.0 |
Converted to Millions | 18.72 |
Despite significant investment, the system still struggles with scalability, speed, efficiency, consistency, and predictive capabilities.
Furthermore, AI can significantly enhance the sensor-to-shooter link in combating terrorism along the Pakistan-Afghan border, where rapid and accurate responses are critical. By integrating AI into surveillance systems, security forces can improve their ability to monitor and interpret vast amounts of data from drones, ground sensors, and other sources. AI algorithms can analyze these inputs in real-time, distinguishing between normal civilian activities and behaviors indicative of terrorist actions, such as unusual cross-border movements or the assembly of suspicious groups. This capability enables faster and more accurate threat assessments, allowing for immediate decisions on how to respond.
Moreover, AI enhances target acquisition, ensuring that counter-terrorism measures are precise and minimize collateral damage, even in complex environments where terrorists may blend in with civilians. Automated and semi-automated response systems, such as AI-controlled drones, can engage threats as soon as they are confirmed, reducing the time between detection and action. Additionally, AI provides valuable decision support by simulating scenarios and predicting outcomes, helping commanders choose the most effective strategies.
However, the use of AI comes with its own drawbacks. AI’s ability to analyze the vast amount of data can make surveillance networks such as cameras more effective and enhance their utility for security purposes. Training AI models to analyze footage produced by cameras is not an easy task, and AI models designed in other countries cannot be useful in Pakistan due to the distinct nature of data and cultural specificities. For example, there are regions of Pakistan where common people and Taliban wear the same attire and carry weapons. Due to these reasons, identifying threats using AI that does not understand these differences, might prove counterproductive.
Due to these cultural similarities, if AI systems only relied on the footage from cameras, they might get confused. Consequently, it might start highlighting civilians as Taliban. To overcome this problem, AI models are required that are trained on quality data. This data should encompass all aspects of people’s daily lives in these regions so that they understand the subtle clues and does not rely on apparent attire. These AI models should be designed with contextual understanding, a key aspect of their training.
Secondly, the reach and affordability of social media platforms make them an attractive destination for terrorist propaganda. These platforms play an important role in spreading terrorist narratives to the masses. Terrorist organizations such as Tehrik-i-Taliban Pakistan (TTP), ISIS, Al Qaeda, Al Shabab, Boko Haram, and the Revolutionary Armed Forces of Colombia (FARC) exploit social media platforms to spread their hateful content against states to influence the general public. Due to sheer volume of content produced on social media sites, manual monitoring and removal becomes nearly impossible.
According to DataReportal’s report, there are 110 million internet users in Pakistan, and 71.70 million active social media users. Let us assume, at a minimum level, that if 71.70 million active users each make one post per day, this would result in 71.70 million posts per day, 2.15 billion posts in one month, and 26.171 billion posts in one year. Let’s assume that 0.5 percent of such posts spread extremism, resulting in 358,500 posts per day, 10.755 million posts per month, and 130 million posts per year. If these extremist posts reach one percent of Pakistani social media users, then they can reach 3,585 people in one day, 107,550 people in one month, and 1.3 million people in one year. Assuming that these posts can influence one percent of the people consuming this content, then in a year, it could influence more than 13,000 people. Even with the most conservative estimates, the presence of such posts online presents a significant threat.
Description | Value |
Active Social Media Users | 71.7 million |
Posts per Day | 71. 70 million |
Posts per Month | 2. 1 billion |
Posts per Year | 26.17 billion |
Assuming 0.5 percent of Posts Spreading Extremist Content (Per Day) | 358500 |
Assuming 0.5 percent of Posts Spreading Extremist Content (Per Month) | 10755000 |
Posts Spreading Extremist Content (Per Year) | 130852500 |
People Consuming Extremist Content (Per Day) | 3585 |
People Consuming Extremist Content (Per Month) | 107550 |
People Consuming Extremist Content (Per Year) | 1308525 |
Monitoring even one percent of the posts available online becomes an overwhelming task for humans. AI tools can automatically remove the extremist content present on social media sites. Utilizing AI tools can be helpful nipping the evil in the bud. These tools can analyze the historical data of social media activities and also have the ability to combine it with other intelligence sources to predict the threat of terrorist activities. These tools can also help in identifying the early signs of radicalization present in an individual or a group.
The role of prediction cannot be underestimated in the prevention of such attacks. Due to the AI’s ability to analyze a sheer volume of data, which is humanly impossible to analyze, it can help in highlighting the patterns of behavior. This can be helpful in finding new threats because instead of traditional investigation methods, which start from the known suspects, it can give investigators the ability to analyze activities of a large population. However, to improve the effectiveness of these models, Pakistani authorities should work to improve the quality of these models using different local languages used in the propaganda of terrorist organizations such as TTP.
In the table below, it is shown how terrorist organizations such as TTP spread their content. The breakdown of terrorist organizations shows that terrorists spread most of their content in three languages such as Urdu, Arabic, and Pashto. They also attempt to publish content in other languages such as English and Balochi.
Language Breakdown of TTP Videos
Language Type | Percentage and Viewers |
Urdu Nasheed | 5% (18m) |
Urdu Speech | 19% (62m) |
Pashto Nasheed | 19% (62m) |
Pashto Speech | 20% (64m) |
Arabic Nasheed | 26% (84m) |
Arabic Speech | 2% (7m) |
Balochi Speech | 1% (2m) |
English Speech | 8% (25m) |
Source: NACTA Journal
The table above shows that TTP produces multilingual content, underscoring the challenge of moderating extremist propaganda and hate speech, where the boundaries are often less clearly defined. Developing tools to accurately detect and remove such content is complex, especially when robust datasets for training algorithms are scarce in less commonly spoken languages. Consequently, harmful content in these languages may spread more easily. Even large social media companies like Facebook, despite having advanced AI models, struggle to detect new extremist content in various languages effectively.
Facebook report that they use AI for content moderation. It claims that 65.4% of hate speech content was found and flagged before user reports,” this statistic can be misleading. Often, what is being identified automatically are merely variations of content previously reviewed by humans, not entirely new instances of inappropriate content. Such statistics may give the impression that machine learning techniques are effectively identifying fresh content, which is not always the case.
Moreover, there is a significant disparity in how social media companies handle content moderation across different languages. For example, while only 29 percent of English-language COVID misinformation was missed by automatic detection on Facebook, almost 70 percent of similar misinformation in Italian and Spanish went unflagged. Moreover, leaked documents have shown that Arabic-language posts are frequently misidentified as hate speech. This lack of effective moderation in local languages has contributed to human rights abuses. To improve AI Models, they should be trained on existing data labeled as hateful. Although social media platforms possess vast amounts of labeled data, the quality of the data can be questionable due to inherent biases and existing policy frameworks. As a result, AI moderation models may lack creativity.
Moreover, mitigating extremist social media content in Pakistan, especially in languages such as Pashto, English, Arabic, and other regional dialects, requires a multi-pronged approach. The effectiveness of these models can be improved through cooperation with social media companies, which can facilitate refining AI models for specific languages. Furthermore, there is a need to adapt current technologies and train these systems on high-quality data that recognizes linguistic uniqueness. Pakistan should develop AI models trained on local data that comprehend the ground realities of Pakistan. These models should be capable to meet local demands and possess contextual understanding. As a result, these models will enhance content moderation capability and proving effective against the extremist content even in local languages. However, success of these models depends on the quality of training data. The availability of data in multiple languages is key in the success of these models.
In conclusion, AI-driven strategies can play a significant role in counter terrorism and violent extremism by enabling the analysis of vast amount of data, identify potential threats, and mitigating risks that are unmanageable by humans. To make AI successful in the context of Pakistan, it is vital to develop local models that incorporate local languages, cultural dynamics, and regional threats. Without AI, fully leveraging current safety projects in Pakistan is not feasible. Due to AI’s ability to manage vast amount of data, the usefulness of these projects can be enhanced. Moreover, terrorists are using social media to spread propaganda. Given the large volumes of content produced on social media platforms, monitoring and stopping it becomes an impossible task. AI can help in analyzing vast datasets and generating patterns. Moreover, AI can facilitate in making sense of that data using different methods of analysis such as behavioral and sentiment analysis.
However, even the current AI models used by social media companies face challenges in analyzing the content in the local languages. Therefore, it is necessary to strengthen partnerships with social media companies to improve their content moderation capabilities. In addition, Pakistan should develop local AI models to combat online violent extremism. To gain the benefits of AI in counter-terrorism strategies, there is a need to improve the quality of available data and incorporate local languages and cultural contexts in AI training models.
The views presented in this article are those of the speaker or author and do not necessarily represent the views of DoD or its components.